Wait, I Can’t Use p < 0.05?
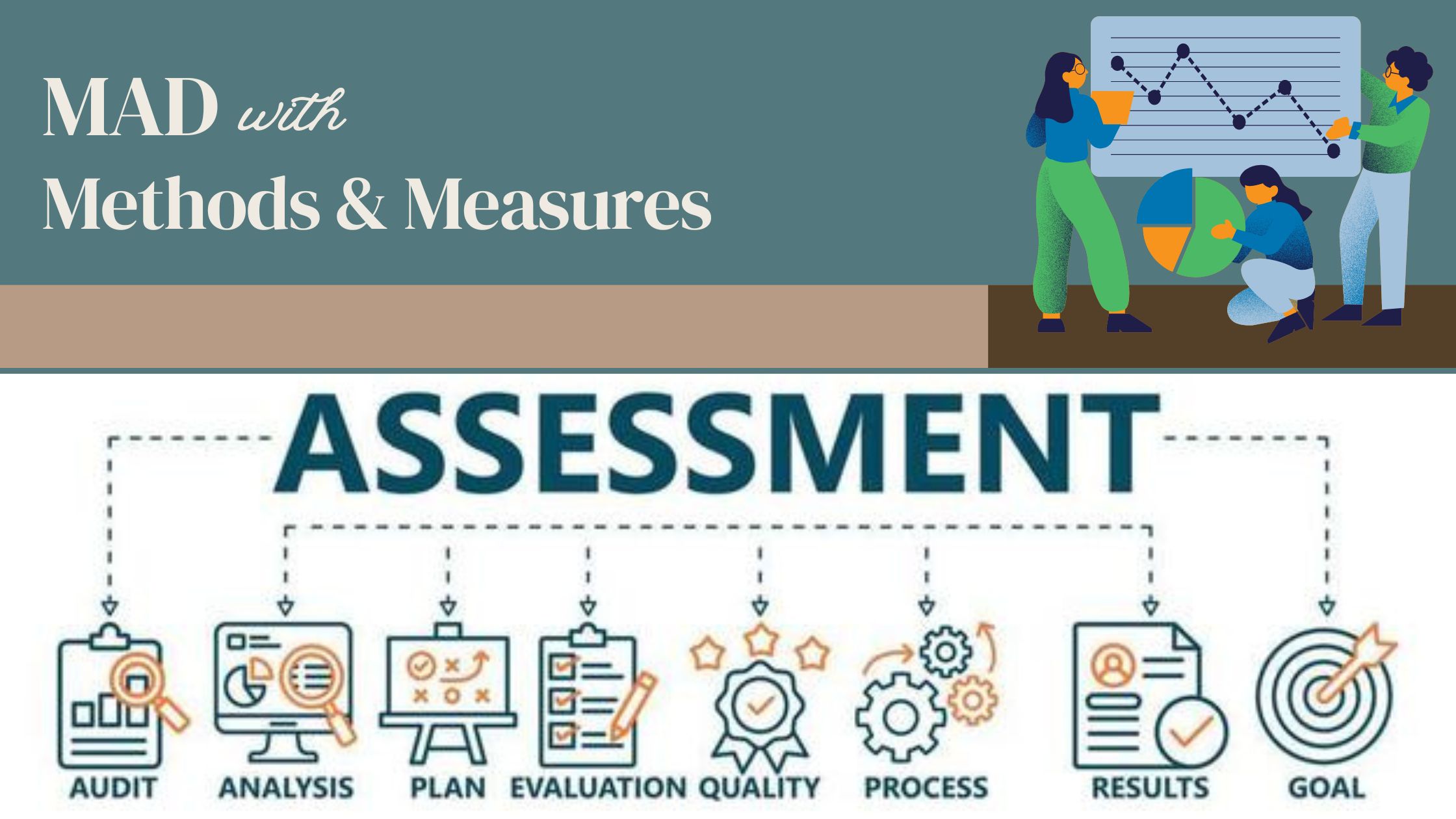
By Jake Working
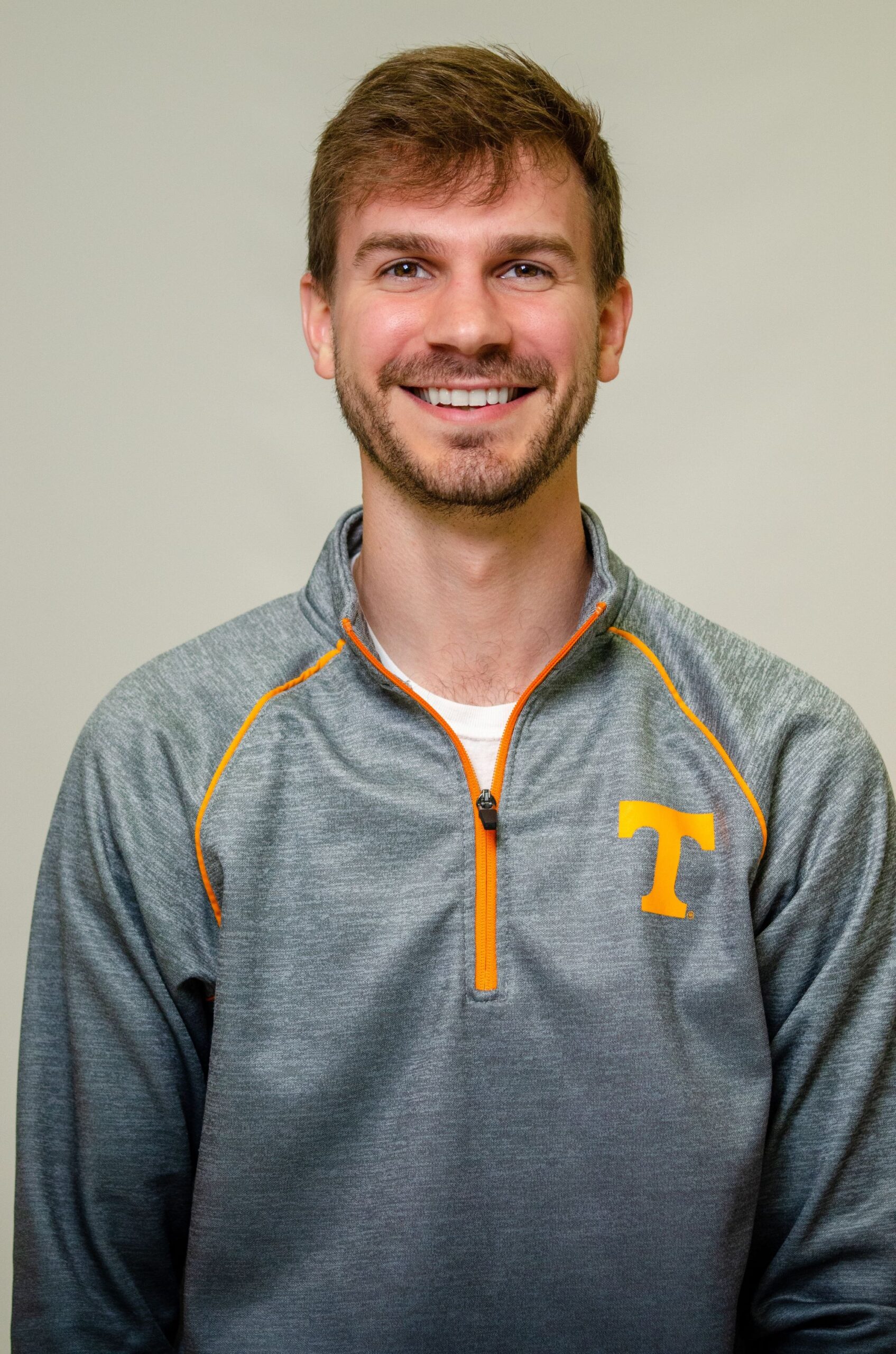
Introduction
You might have heard the recent rumblings in the statistics world: null hypothesis significance testing, statistical significance, p-values, our beloved p-value, have been coming into question. Well, the statistical soundness of these methods is not being doubted, but their current use and interpretations in applied research have been.
How did we get here? Why are interpretations of significance testing and p-values under fire? What does this mean for you, the applied researcher who uses these methods?
The literature surrounding this topic is huge, so I will start to provide some background to these questions in this blog post by including a brief introduction to a few important articles. My name is Jake Working, and I am currently studying for my Ph.D. in Evaluation, Statistics, and Methodology at the University of Tennessee, Knoxville. Let’s learn together.
How Did We Get Here?
Understanding the history of null hypothesis significance testing and p-values is just as important as crafting the future of these analytical methods. In this section, I direct you to check out Lee Kennedy-Shaffer’s article “Before p < 0.05 to Beyond p < 0.05: Using History to Contextualize p-Values and Significance Testing” (2019).
Kennedy-Shaffer reminds us of the history of significance testing and the p-value, noting Sir Ronald Fisher’s popularization of p < 0.05 through historical and contextual lens. Fisher was advancing statistical methodology at the same time as statistic legends such as Karl Pearson (yes, that Pearson) and William Gosset (of Guinness “student” fame), who were all developing uses for significance testing. Fisher formed his suggested p < 0.05 as a simple cut-off of significance in 1925. His reasoning was simple: “p = 0.05, or 1 in 20, is 1.96 or nearly 2…deviations exceeding twice the standard deviation are thus formally regarded as significant” (Fisher, 1925, p. 47 in Kennedy-Shaffer, 2019, p. 84).
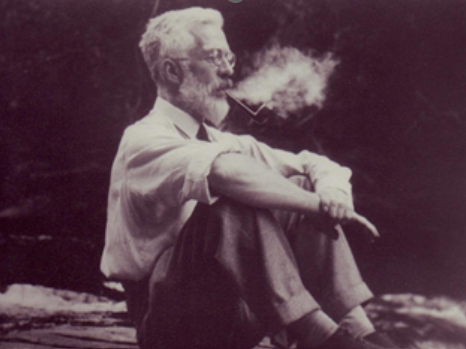
Sir Ronald Fisher, circa 1946, thinking about p-values,
from University of Adelaide (source)
Criticisms and alternatives to interpretations to significance testing have existed since the onset of null hypothesis significance testing. These include Neyman-Pearson’s alpha (1933), Bayes’ inverse probability, and Fisher himself even challenged the field against a fixed level of significance (Kennedy-Shaffer, 2019, pp. 85-86). So, what’s the beef with p-values now?
Laying Down the Law
As the discussion on p-values and other flaws in statistical reporting seemed to rekindle in the mid-2010s, the American Statistical Association decided to provide the scientific and research community with grounded direction on p-values. In this section, I urge you to read the very short, but impactful “ASA Statement on p-Values: Context, Process, and Purpose” by Ronald Wasserstein and Nicole Lazar (2016).
They articulated six simple principles on p-values:
- P-values can indicate how incompatible the data are with a specified statistical model
- P-values do not measure the probability that the studied hypothesis is true, or the probability that the data were produced by random chance alone
- Scientific conclusions and business or policy decisions should not be based only on whether a p-value passes a scientific threshold
- Proper inference requires full reporting and transparency
- A p-value, or statistical significance, does not measure the size of an effect or the importance of a result
- By itself, a p-value does not provide a good measure of evidence regarding a model or hypothesis
These principles urge the researcher to contextualize and completely understand their data and analysis methods, making useless bright lines such as p < 0.05. Rosnow and Rosenthal (1989) said it neatly: “…surely, God loves the .06 nearly as much as the .05” (p. 1277).
Okay, so what do I do now?
If you are a researcher, student, or just interested in statistical analysis, one thing you can do is to update your analytical habits. Check out this article by Wasserstein, Lazar, and Schirm: “Moving to a World Beyond ‘p < 0.05’” (2019) for context and suggestions.
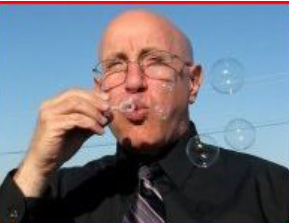
Another Ronald, Ron Wasserstein, doing his best Fisher imitation,
from Amstat News (source)
Included in their article is a mental framework to guide future use of these statistical methods they summarize into two sentences: “Accept uncertainty. Be thoughtful, open, and modest” (Wasserstein et al., 2019, p. 2). Their framework is helpful to set your mental state before delving into the eight pages of action items summarized from 43 different articles on this topic.
Wasserstein et al. make it an easy read by summarizing each article into actionable bullet points and organizing the suggestions into five topic areas:
- Getting to a Post “p < 0.05” Era
- Interpreting and Using p
- Supplementing or Replacing p
- Adopting More Holistic Approaches
- Reforming Institutions: Changing Publication Policies and Statistical Education
Call to Action
As it would be impossible to summarize everything from these articles into one blog post, I urge you to read the three articles in this post. You will better understand p-values and become a better researcher, evaluator, and statistician because of it.
- “Before p < 0.05 to Beyond p < 0.05: Using History to Contextualize p-Values and Significance Testing” (Kennedy-Shaffer, 2019)
- “The ASA Statement on p-Values: Context, Process, and Purpose” (Wasserstein & Lazar, 2016)
- “Moving to a World Beyond p < 0.05” (Wasserstein et al., 2019)
No need to abandon hypothesis testing and p-values, but be prepared to better understand these tools for what they are: statistical tools.
References
Kennedy-Shaffer L. (2019). Before p < 0.05 to Beyond p < 0.05: Using History to Contextualize p-Values and Significance Testing. The American Statistician, 73(Suppl 1), 82–90. https://doi.org/10.1080/00031305.2018.1537891
Rosnow, R.L. & Rosenthal, R. (1989). Statistical procedures and the justification of knowledge in psychological science. American Psychologist, 44, 1276-1284.
Wasserstein, R. L., & Lazar, N. A. (2016). The ASA Statement on p-Values: Context, Process, and Purpose. The American Statistician, 70(2), 129-133. https://doi.org/10.1080/00031305.2016.1154108
Wasserstein, R. L., Schirm, A. L., & Lazar, N. A. (2019). Moving to a World Beyond “p< 0.05”. The American Statistician, 73(sup1), 1-19. https://doi.org/10.1080/00031305.2019.1583913